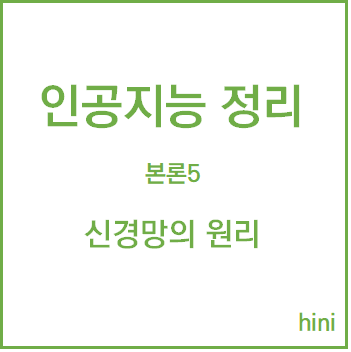
์ ๊ฒฝ๋ง์ ์๋ฆฌ ์ด์ multi-layer perceptron์ ์ด์ฉํ ์ ์๊ฒ ๋์์ง๋ง, ์ ํํ ๊ตฌ๋์ํค๊ธฐ ์ํด ์์์ผํ ๊ฒ์ด ์๋ค. ์ฃผ์ด์ง input์ ๊ฐ์ง๊ณ ๊ณ์ฐ์ ํด์ output์ ๋ผ ์๋ ์์ง๋ง, output ๊ฐ์ด ์ฃผ์ด์ง ์ ๋ต๊ณผ ๋ค๋ฅผ ๋ ํ์ตํ๋ ๊ธฐ๋ฅ์ ์์ง ๊ตฌํํ์ง ๋ชปํ๋ค. ์ฌ๊ธฐ์ ํ์ต์ด๋ w๊ฐ์ ์กฐ์ ํ๋ ๊ฒ์ ๋งํ๋ค. w๊ฐ์ ์กฐ์ ํ๋ ํ์ต์ ํ๊ธฐ ์ํด์๋ ๋๋ค, ์กฐ๊ธ์ฉ ์ฎ๊ฒจ๋ณด๊ธฐ ์ ๋ฐฉ์๋ณด๋ค๋ ๋ฏธ๋ถ์ ์ฌ์ฉํ๋ ๊ฒ์ด ์ข๋ค. w์ ๋ํ Error์ ๋ฏธ๋ถ๊ฐ์ ๊ตฌํ ํ ๋ฐ๋์ชฝ์ผ๋ก ์ผ์ ์น๋งํผ ์์ง์ฌ์ผ ํ๋ฏ๋ก Error๋ฅผ w์ ์์ผ๋ก ๋ํ๋ผ ์ ์์ด์ผ ํ๊ณ , ์กฐ์ ๋๋ w์ ๊ฐ์ ์ด์ ๊ด๋ จํ์ฌ ํํํ ์ ์์ด์ผํ๋ค. ๊ฒฐ๊ตญ error๋ฅผ ์ค์ด๊ธฐ ์ํด w๊ฐ์ ์กฐ์ ํ๋ ๊ณผ์ ์ error์ ๋ํ w์ ๋ฏธ๋ถ๊ฐ์ด ํ์..
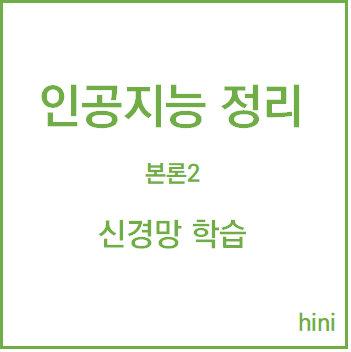
์ ๊ฒฝ๋ง ํ์ต ์ธ๊ฐ์ ๋์ธํฌ ๋ด๋ฐ์ ๋ณธ๋ด classifier Collection => Processing => Dissemination(์ ํ) ๊ณผ์ ์ ๊ฑฐ์นจ ๋จ์ผ ์ ๊ฒฝ๋ง ๊ธฐ๋ณธ ๊ตฌ์กฐ ๋ด๋ฐ : input(์ ๋ ฅ), weight(ํ์ต, ๊ธฐ์ต), net(ํ์ฑํ ๋ ๋ฒจ), ํ์ฑํ ํจ์ ๋ถ์ ๋ด๋ฐ์ weight๋ ํ์ต, ๊ธฐ์ต์ ์ญํ ์ ํจ ( ==๋ด๋ฐ์ ๊ธฐ๋ฅ ) ๋ณ๊ฒฝ๋๋ฉด ๋ด๋ฐ์ ๊ธฐ๋ฅ์ด ๋ณ๊ฒฝ๋จ x [input] * w [weight] = net O = f(net) [activation function] Error = T [answer] - O [f(net)] ์ด๋ฌํ ํ๋ฆ์ผ๋ก ๊ณ์ฐํ๋ ๊ฒ์ forward propagation์ด๋ผ๊ณ ํจ ๋ชฉํ : input์ ๋ฐ๋ฅธ output์ด ์ ํํ ๋ถ๋ฅ๋์ด ๋์์ผ ํจ Error๊ฐ 0์ ๊ฐ๊น์์ง..
Comment